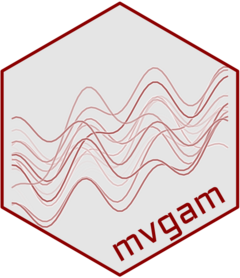
Helper functions for marginaleffects calculations in mvgam models
Source:R/marginaleffects.mvgam.R
mvgam_marginaleffects.Rd
Helper functions for marginaleffects calculations in mvgam models
Functions needed for working with marginaleffects
Functions needed for getting data / objects with insight
Usage
# S3 method for mvgam
get_coef(model, trend_effects = FALSE, ...)
# S3 method for mvgam
set_coef(model, coefs, trend_effects = FALSE, ...)
# S3 method for mvgam
get_vcov(model, vcov = NULL, ...)
# S3 method for mvgam
get_predict(model, newdata, type = "response", process_error = FALSE, ...)
# S3 method for mvgam
get_data(x, source = "environment", verbose = TRUE, ...)
# S3 method for mvgam_prefit
get_data(x, source = "environment", verbose = TRUE, ...)
# S3 method for mvgam
find_predictors(
x,
effects = c("fixed", "random", "all"),
component = c("all", "conditional", "zi", "zero_inflated", "dispersion", "instruments",
"correlation", "smooth_terms"),
flatten = FALSE,
verbose = TRUE,
...
)
# S3 method for mvgam_prefit
find_predictors(
x,
effects = c("fixed", "random", "all"),
component = c("all", "conditional", "zi", "zero_inflated", "dispersion", "instruments",
"correlation", "smooth_terms"),
flatten = FALSE,
verbose = TRUE,
...
)
Arguments
- model
Model object
- trend_effects
logical
, extract from the process model component (only applicable if atrend_formula
was specified in the model)- ...
Additional arguments are passed to the
predict()
method supplied by the modeling package.These arguments are particularly useful for mixed-effects or bayesian models (see the online vignettes on themarginaleffects
website). Available arguments can vary from model to model, depending on the range of supported arguments by each modeling package. See the "Model-Specific Arguments" section of the?slopes
documentation for a non-exhaustive list of available arguments.- coefs
vector of coefficients to insert in the model object
- vcov
Type of uncertainty estimates to report (e.g., for robust standard errors). Acceptable values:
FALSE: Do not compute standard errors. This can speed up computation considerably.
TRUE: Unit-level standard errors using the default
vcov(model)
variance-covariance matrix.String which indicates the kind of uncertainty estimates to return.
Heteroskedasticity-consistent:
"HC"
,"HC0"
,"HC1"
,"HC2"
,"HC3"
,"HC4"
,"HC4m"
,"HC5"
. See?sandwich::vcovHC
Heteroskedasticity and autocorrelation consistent:
"HAC"
Mixed-Models degrees of freedom: "satterthwaite", "kenward-roger"
Other:
"NeweyWest"
,"KernHAC"
,"OPG"
. See thesandwich
package documentation.
One-sided formula which indicates the name of cluster variables (e.g.,
~unit_id
). This formula is passed to thecluster
argument of thesandwich::vcovCL
function.Square covariance matrix
Function which returns a covariance matrix (e.g.,
stats::vcov(model)
)
- newdata
Grid of predictor values at which we evaluate the slopes.
Warning: Please avoid modifying your dataset between fitting the model and calling a
marginaleffects
function. This can sometimes lead to unexpected results.NULL
(default): Unit-level slopes for each observed value in the dataset (empirical distribution). The dataset is retrieved usinginsight::get_data()
, which tries to extract data from the environment. This may produce unexpected results if the original data frame has been altered since fitting the model.datagrid()
call to specify a custom grid of regressors. For example:newdata = datagrid(cyl = c(4, 6))
:cyl
variable equal to 4 and 6 and other regressors fixed at their means or modes.See the Examples section and the
datagrid()
documentation.
subset()
call with a single argument to select a subset of the dataset used to fit the model, ex:newdata = subset(treatment == 1)
dplyr::filter()
call with a single argument to select a subset of the dataset used to fit the model, ex:newdata = filter(treatment == 1)
string:
"mean": Slopes evaluated when each predictor is held at its mean or mode.
"median": Slopes evaluated when each predictor is held at its median or mode.
"balanced": Slopes evaluated on a balanced grid with every combination of categories and numeric variables held at their means.
"tukey": Slopes evaluated at Tukey's 5 numbers.
"grid": Slopes evaluated on a grid of representative numbers (Tukey's 5 numbers and unique values of categorical predictors).
- type
string indicates the type (scale) of the predictions used to compute contrasts or slopes. This can differ based on the model type, but will typically be a string such as: "response", "link", "probs", or "zero". When an unsupported string is entered, the model-specific list of acceptable values is returned in an error message. When
type
isNULL
, the first entry in the error message is used by default.- process_error
logical
. IfTRUE
, uncertainty in the latent process (or trend) model is incorporated in predictions- x
A fitted model.
- source
String, indicating from where data should be recovered. If
source = "environment"
(default), data is recovered from the environment (e.g. if the data is in the workspace). This option is usually the fastest way of getting data and ensures that the original variables used for model fitting are returned. Note that always the current data is recovered from the environment. Hence, if the data was modified after model fitting (e.g., variables were recoded or rows filtered), the returned data may no longer equal the model data. Ifsource = "frame"
(or"mf"
), the data is taken from the model frame. Any transformed variables are back-transformed, if possible. This option returns the data even if it is not available in the environment, however, in certain edge cases back-transforming to the original data may fail. Ifsource = "environment"
fails to recover the data, it tries to extract the data from the model frame; ifsource = "frame"
and data cannot be extracted from the model frame, data will be recovered from the environment. Both ways only returns observations that have no missing data in the variables used for model fitting.- verbose
Toggle messages and warnings.
- effects
Should model data for fixed effects (
"fixed"
), random effects ("random"
) or both ("all"
) be returned? Only applies to mixed or gee models.- component
Which type of parameters to return, such as parameters for the conditional model, the zero-inflated part of the model, the dispersion term, the instrumental variables or marginal effects be returned? Applies to models with zero-inflated and/or dispersion formula, or to models with instrumental variables (so called fixed-effects regressions), or models with marginal effects (from mfx). See details in section Model Components .May be abbreviated. Note that the conditional component also refers to the count or mean component - names may differ, depending on the modeling package. There are three convenient shortcuts (not applicable to all model classes):
component = "all"
returns all possible parameters.If
component = "location"
, location parameters such asconditional
,zero_inflated
,smooth_terms
, orinstruments
are returned (everything that are fixed or random effects - depending on theeffects
argument - but no auxiliary parameters).For
component = "distributional"
(or"auxiliary"
), components likesigma
,dispersion
,beta
orprecision
(and other auxiliary parameters) are returned.
- flatten
Logical, if
TRUE
, the values are returned as character vector, not as list. Duplicated values are removed.
Value
Objects suitable for internal 'marginaleffects' functions to proceed.
See marginaleffects::get_coef()
, marginaleffects::set_coef()
,
marginaleffects::get_vcov()
, marginaleffects::get_predict()
,
insight::get_data()
and insight::find_predictors()
for details